Business
The billionaire pipeline: How a 2000s startup boom fueled Europe’s tech renaissance
Published
1 week agoon
By
Jace Porter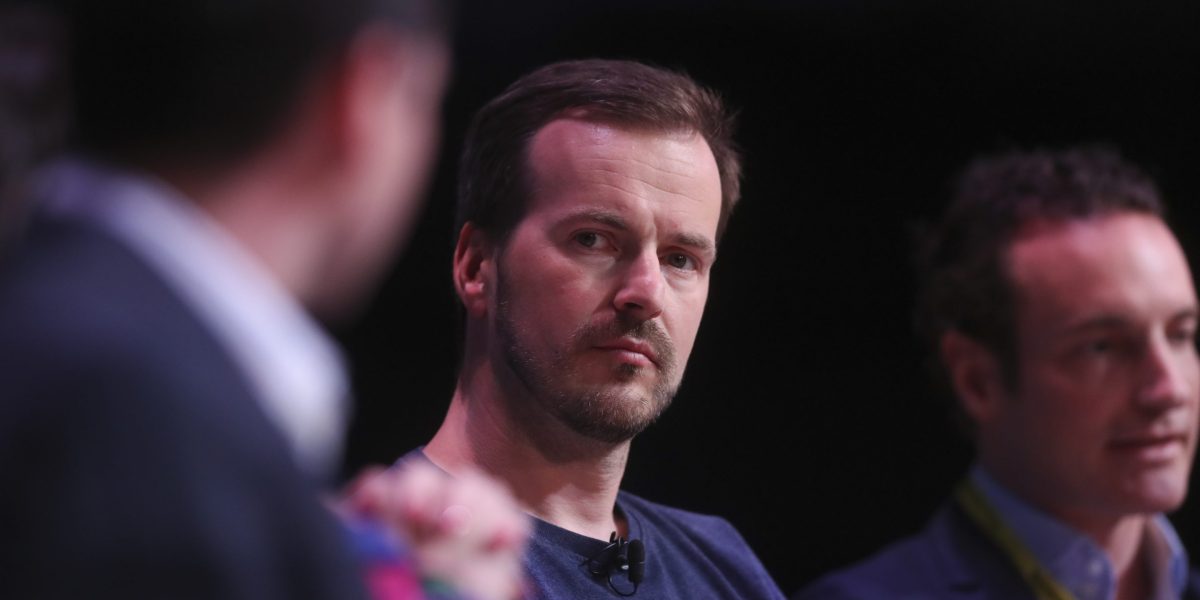
Picture the scene. It’s 2003 in Tallinn, Estonia. Taavet Hinrikus, a 20-year-old computer whiz, has just accepted an offer to become the first employee of a little-known video-call startup, Skype. Little did he know then, but it was the start of a multi-decade evolution that would make him one of Estonia’s first billionaires, spawn dozens of startups, and generate billions of dollars in venture funding across Europe. “The idea that you can start using this [video-chat technology] to have voice and video conversations was pretty crazy for a boy who was born in the Soviet Union. But it was also obvious to me that it was going to be an exciting journey,” Hinrikus tells Fortune.
Today, at age 43, Hinrikus can add cofounder of payment platform Wise and partner of founder-led venture capital fund Plural Platform to his résumé, having steered multiple companies through countless funding rounds. Hinrikus’s story—unique at the turn of the century—would make him a pioneer in a fascinating business trend: In Europe, a surprisingly high number of employees of unicorns like Skype would go on to launch still more unicorns.
Europe’s founder factories
It would be an understatement to say that Europe’s tech scene has undergone a revolution since Hinrikus started his first day at Skype.
“The idea that you can start using this [video-chat technology] to have voice and video conversations was pretty crazy for a boy who was born in the Soviet Union…”
Taavet Hinrikus
Some 1,650 European tech startups have been founded across Europe by former employees of 215 unicorns since 2008, according to data provided by venture capital group Accel and Dealroom.co. The pair provided Fortune with data on European spin-outs of startups in the region, having separately analyzed Europe and Israel starup activity over the21st century.
Certain countries have punched above their weight. Sweden, for example, is a standout performer, breeding the multibillion-dollar businesses Spotify and Klarna. Employees from that pair have founded a further 123 startups. King.com, the Swedish gaming group behind Candy Crush Saga, has seen 43 employees leave to create their own companies.
Skype employees would go on to launch 31 startups in total, including Hinrikus’s Wise and the ride-hailing group Bolt. To date, those startups have raised $3.5 billion in funding.
1,650
The number of European tech startups founded across Europe since 2008.
Most founders, around 55%, start their businesses in the same European city where they were first employed. This has helped spawn network effects across Europe that have turned unlikely cities, like Tallinn, into thriving tech hubs.
Repeat founders have also blossomed from the early-2000s scene. Spotify cofounder and CEO Daniel Ek, perhaps the most high-profile founder to emerge from Europe this century, recently announced a new funding round at a $1.7 billion valuation for his health-tech startup, Neko Health, making him a serial unicorn creator.
The question is, why did it take Europe so long to kick-start its entrepreneurial streak? And what changed to allow the continent’s founder factories to flourish?
Trailblazers
When Netherlander Harry Nelis—a partner at American fund Accel—who has operated out of Europe for the past 21 years, interviews a candidate for his company, he always asks the same question: “What’s the riskiest thing that you’ve ever done in your life?”
Nelis’s own answer? Getting married (he says he’s been happily married for 30 years now). But a close second might be Spotify. Nelis was part of the team that gave Spotify early financial backing, despite industry experts warning that a streaming music business would never work.
“The momentum was almost undeniable,” Nelis recalls when asked why he backed it anyway. “The product was so good and so easy to use, and the early consumer reaction so overwhelming, the company actually had a chance to make it.”
Think of any multibillion-dollar European tech company today, and it’s likely Accel was involved in its inception. After the group raised Series A funding for U.S. companies like Facebook, Nelis’s only real mandate in Europe was to find entrepreneurs with “big ideas.” That daunting brief is probably why he still asks job candidates about risk today.
“The biggest mistake in venture is not losing money on an investment. It is missing the outlier,” Nelis tells Fortune from Accel’s London office.
When he first came back to Europe after spending his early career in Silicon Valley, Nelis was struck by an obvious difference in attitude between Americans and Europeans, namely that it was unusual for the latter to pursue building a company instead of joining an established one.
“The biggest mistake in venture is not losing money on an investment. It is missing the outlier.”
Harry Nelis, partner at Accel
Europe has long been accused of lacking the work ethic often associated with Americans. Tom Blomfield, cofounder of British unicorns GoCardless and Monzo, last year accused the U.K. of suffering from a “know your place” attitude that suppressed entrepreneurship.
Matt Robinson, a fellow GoCardless cofounder and now a partner at Accel, disagrees with that assessment. However, like his colleague Nelis, Robinson did notice a difference in Europeans’ attitude toward entrepreneurship when he started GoCardless in 2011.
“Starting a company was not really an accepted thing to do. You know, when you sit over here and start a company, I think people assume you’re unemployed or unemployable,” he says.
Some elements crucial to growing a startup, like access to seed funding, were nascent in the U.K. just 15 years ago, Robinson notes.
Those who spoke to Fortune for this article, though, were aligned in their assessment that rather than an attitude overhaul, Europe just needed a few successful founders to show everyone else what was possible.
Ilkka Paananen, CEO and co-founder of Finnish mobile gaming unicorn Supercell, was one of those entrepreneurs working without a roadmap to follow.
“There were very few European tech entrepreneurs who I could call for advice, for the simple reason that we just did not have many tech startups at scale at that time,” Paananen recalled.
Nelis says Europe’s startup founders were role models who made success easier to envision for their successors. One of those would be Plural’s Hinrikus, who watched Skype become one of Europe’s first unicorns.
“People know the drill better,” Nelis says, noting that new startups come to Accel today with plans to solve big problems in a way they often didn’t 20 years ago.
Plural’s Hinrikus says his crystallizing moment came when he realized Niklas Zennström, cofounder of Skype, didn’t possess any magical powers that made him more likely to be a successful founder: “He was an average person, just like me. If he can do it, then I can equally do it.”
Robinson says the major obstacles in building a startup became easier for him the second time around, namely, attracting the best talent and fundraising.
Since Nelis returned to Europe in 2004, unicorns and decacorns have emerged from Europe’s VC pipeline, with a centacorn surely inevitable. Robinson spoke to one company that talked ambitiously about becoming the first-ever kilocorn, a $1 trillion private startup.
“I cannot imagine saying that or even thinking that back in 2011,” Robinson says.
Stick or twist?
Operating a thriving entrepreneurial startup environment brings the inherent and evidenced risk that employees will one day leave to start their own, sometimes competing, ventures.
Tara Ryan, Monzo’s VP of people experience, doesn’t see it as a tradeoff.
Monzo stands among Europe’s most prolific founder factories. The banking unicorn has spawned 23 startups since its creation. Oftentimes, when a new company is formed out of Monzo, it’s not just one person departing.

“People start their own businesses, but often their founding team or their first handful of employees are also Monzonauts,” she says.
This has been something embraced, rather than suppressed. At Monzo, Ryan says, an internal company website celebrates former employees who went on to become founders.
“People start their own businesses, but often their founding team or their first handful of employees are also Monzonauts.”
Tara Ryan, Monzo’s VP
“I don’t think it is healthy for employees or employers to try and retain people at all costs,” she says.
Accel’s Robinson goes one further. While at GoCardless, he would tell early interviewees his hope for them was that they would eventually leave and form their own startups.
The European dream
It’s worth a wager that Hinrikus, dressed in a hoodie and branded tee, and speaking from Plural’s London office, looks as invigorated as he did when he stumbled through Skype’s doors on his first day as an employee.
Skype was acquired by eBay in 2005 for $2.6 billion, a now-familiar case of an exciting European startup being eaten up by a much larger U.S. tech behemoth. Its subsequent parent, Microsoft, no longer needs Skype now that Microsoft’s Teams video-chat function has been widely adopted. Similarly, DeepMind, the pioneering artificial intelligence research laboratory founded in London, is today a Google subsidiary.
Increasingly, though, European companies, driven by growing access to both capital and talent, are managing to stand on their own two feet.
Spotify, Accel’s risky early bet in Europe, had a market value of nearly $125 billion at the beginning of March. Its Scandinavian leadership team has maintained its grasp on the company’s operations as Spotify battles with Apple and Amazon.
Other younger companies across Europe, like Monzo, now face the challenge of growing while maintaining what made them unique as startups. Alex Norström, Spotify’s copresident and chief business officer, has advice for startups on that journey.
“We’ve tried to maintain our entrepreneurial energy as we’ve scaled globally,” he says. “At Spotify, it’s always been about having big ambitions and delivering on them.”
Supercell’s Paananen, meanwhile, thinks Europe’s quirks make it easier for founders to stay true to their roots.
“Europe has a very unique, diverse culture, and a unique way of life that we all love — this is a great place to live and grow a family. This should help us both retain and attract the best talent,” said Paananen.
Hinrikus spoke of making the American Dream come true for his employees, only in Europe.
“I think the scar tissue we have from owning our companies and building them makes us better partners for the next generation,” Hinrikus says. “There’s probably 100 early employees in various positions, even in customer support, who earned a million dollars from stock options.
“Now we’re showing time and time again that it’s not an American Dream,” he notes. “We have the same thing in Europe.”
This article appears in the April/May 2025 issue of Fortune with the headline “The European dream.”
This story was originally featured on Fortune.com
Source link
You may like
Business
Buy-now-pay-later installment plans will now appear on your credit report
Published
31 minutes agoon
April 4, 2025By
Jace Porter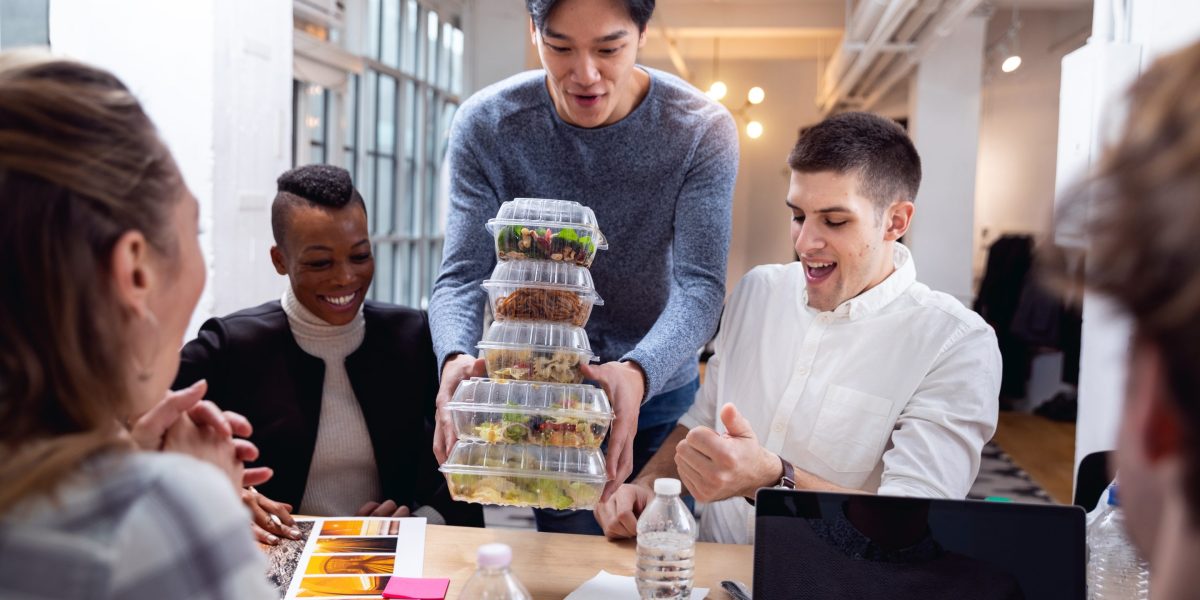
© 2025 Fortune Media IP Limited. All Rights Reserved. Use of this site constitutes acceptance of our Terms of Use and Privacy Policy | CA Notice at Collection and Privacy Notice | Do Not Sell/Share My Personal Information
FORTUNE is a trademark of Fortune Media IP Limited, registered in the U.S. and other countries. FORTUNE may receive compensation for some links to products and services on this website. Offers may be subject to change without notice.
Business
Analysts unanimously denounce Trump’s liberation day tariffs: ‘Worse than the worst case’
Published
1 hour agoon
April 4, 2025By
Jace Porter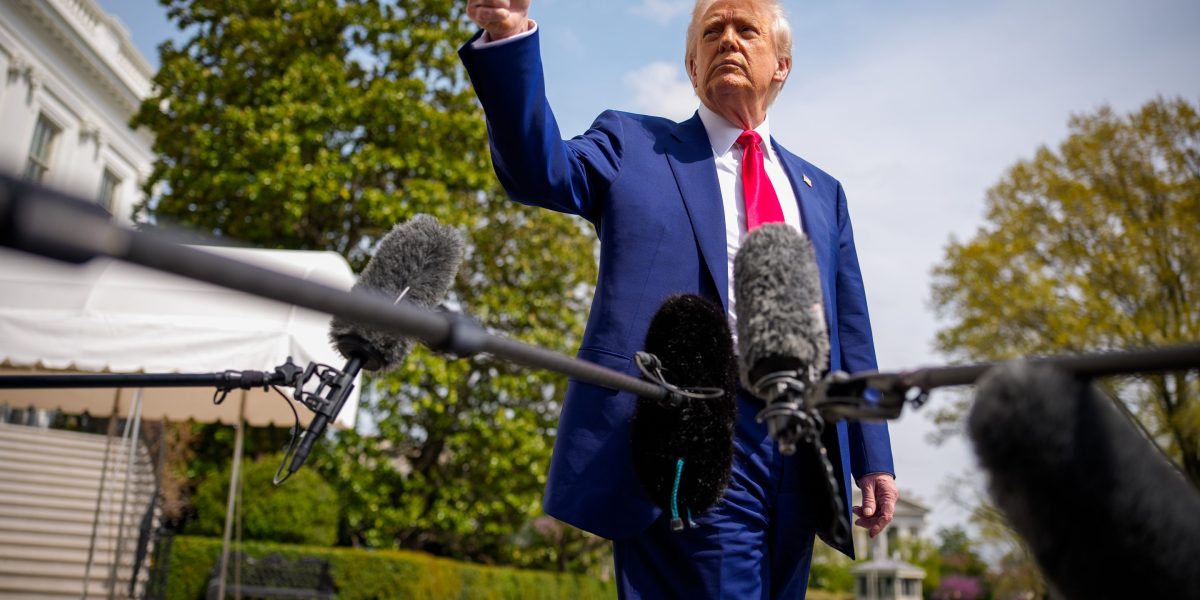
- Respected economists and analysts across the board predicted President Trump’s so-called “Liberation Day” tariffs would have a major effect on the U.S. and world economies. Analysts agreed that the announcement was worse than market onlookers expected as the stock market plummeted Thursday in response.
It’s a truism that economists can’t agree on anything—but President Donald Trump’s so-called “Liberation Day” tariffs have analysts in rare agreement that major economic pain is coming, and it’s worse than any of them predicted. Indeed, it could be even “worse than the worst case.”
During a Wednesday speech at the White House, Trump announced a baseline 10% tariff on imports from all countries and revealed soaring tariffs, which he called “reciprocal,” on some of the United States’ biggest trading partners, including China, Japan, and the European Union.
The announcement sent markets tumbling, with all major indexes down Thursday afternoon. The benchmark S&P 500 was down 4.6% and the Dow Jones was down about 3.7%, but the tech-heavy Nasdaq, which in recent years has guided the stock market to new highs, led the losses with a 5.6% drop.
In response, a slew of respected economists and analysts from major global banks sounded the alarm on a potential recession and predicted changes to the world economic order.
Here are some of the most pointed takes from analysts as they try to make sense of the impending changes.
Wedbush Securities: “Worse than the worst case scenario”
Among the most dismayed was Dan Ives of Wedbush Securities. In a note from Wednesday afternoon, the veteran tech analyst and his team said Trump’s tariffs were even worse than expected.
“President Trump just finished his tariff speech at the White House and we would characterize this slate of tariffs as ‘worse than the worst case scenario’ the Street was fearing,” the analysts wrote.
Ives and his team added that the tariffs on China and Taiwan would especially weigh on technology, and the supply chains of the world’s biggest companies would suffer. The analysts called out Apple, which produces most of its iPhones in China, and Nvidia, which has significant exposure to Taiwan’s semiconductor industry.
In a follow-up note on Thursday, Ives’ team took a shot at the Trump administration’s calculations for the tariffs, calling them “illogical and absurd.”
“If a 9th grader in high school presented this tariff chart to a teacher in a basic economics class the teacher would laugh and say sit down and work on the assignment,” they wrote.
The Wedbush analysts suggested that the absurdity of the numbers show that the tariff rates couldn’t possibly be final and that deals with trading partners are likely to follow. If not, stagflation, the deadly combination of low growth and high inflation, would follow quickly, they wrote.
“Over the coming 24 hours the world will quickly realize these tariff rates will never stay as they are shown otherwise it would be a self-inflicted Economic Armageddon that Trump would send the US and world through over the coming year,” the analysts wrote.
Larry Summers goes after Trump
Former Treasury Secretary Larry Summers took a shot at Trump in a series of posts following the president’s tariff announcement Wednesday.
The respected economist wrote that Trump’s speech cost many Americans real money.
“Never before has an hour of Presidential rhetoric cost so many people so much. Markets continue to move after my previous tweet. The best estimate of the loss from tariff policy is now is closer to $30 trillion or $300,000 per family of four,” Summers wrote in a Wednesday post.
In later posts, Summers also criticized the Trump administration’s calculations, saying they made no sense.
“This is to economics what creationism is to biology, astrology is to astronomy, or RFK thought is to vaccine science,” Summers wrote. “The Trump tariff policy makes little sense EVEN if you believe in protectionist mercantilist economics.”
Finally, Summers—who headed the Treasury under President Bill Clinton—wrote that the tariff announcement was so bad, he would not have tolerated it were he in a government position.
“If any administration of which I was a part had launched an economic policy so totally ungrounded in serious analysis or so dangerous and damaging, I would have resigned in protest,” he added.
Goldman Sachs: Hardware companies to increase prices
Analysts at Goldman Sachs noted that Wednesday’s tariffs were higher than expected and that they would have an effect on hardware companies—even if negotiations ultimately bring those tariff rates lower.
“The magnitude of the tariffs announced is much higher and broader than anticipated by us and investors, and while many might argue that changes could occur through negotiations over the coming days and months, if sustained, the magnitude of the tariff would offer limited options for hardware companies to adjust their supply chains or wait out the term of the current administration,” they wrote in a Thursday note.
The Goldman analysts predicted that because of the breadth of the tariffs, which affect all countries, “price increases to offset the headwinds will be more than just modest.”
The analysts predicted a 5% increase in prices for hardware companies, but some companies with a larger reliance on hardware would take the biggest revenue hits of more than 50%, including information technology company Supermicro, broadband and software company Calix, and optical material and semiconductor manufacturer Coherent.
Oxford Economics: “a global recession will likely be avoided”
One of the rare, slightly upbeat notes came from economic advisory firm Oxford Economics, whose analysts wrote that a recession may not be on the horizon.
“The implementation of the US ‘Liberation Day’ tariff hikes will have a huge impact on individual sectors and firms and will further dampen sentiment. However, our initial assessment suggests a global recession will likely be avoided,” analysts from the firm wrote.
Still, U.S. imports could fall by 15% in three years due to the reciprocal tariffs, which could hit global GDP by 0.5 percentage points this year and 1 percentage point in 2026, the analysts said.
They also believe that any hopes of lessened uncertainty following the Wednesday announcement were unfounded. Even if countries were able to negotiate tariffs down lower or to nothing, the process would still be long.
“One hope is that deals will be struck quickly, meaning that tariff hikes are partially or fully reversed. While such an outcome is possible, tariffs typically rise quickly but fall slowly. With many economies subject to individual reciprocal tariffs, governments may face a lengthy wait before they can even enter negotiations,” the analysts wrote.
This story was originally featured on Fortune.com
Source link
Business
Stop chasing AI benchmarks—create your own
Published
2 hours agoon
April 4, 2025By
Jace Porter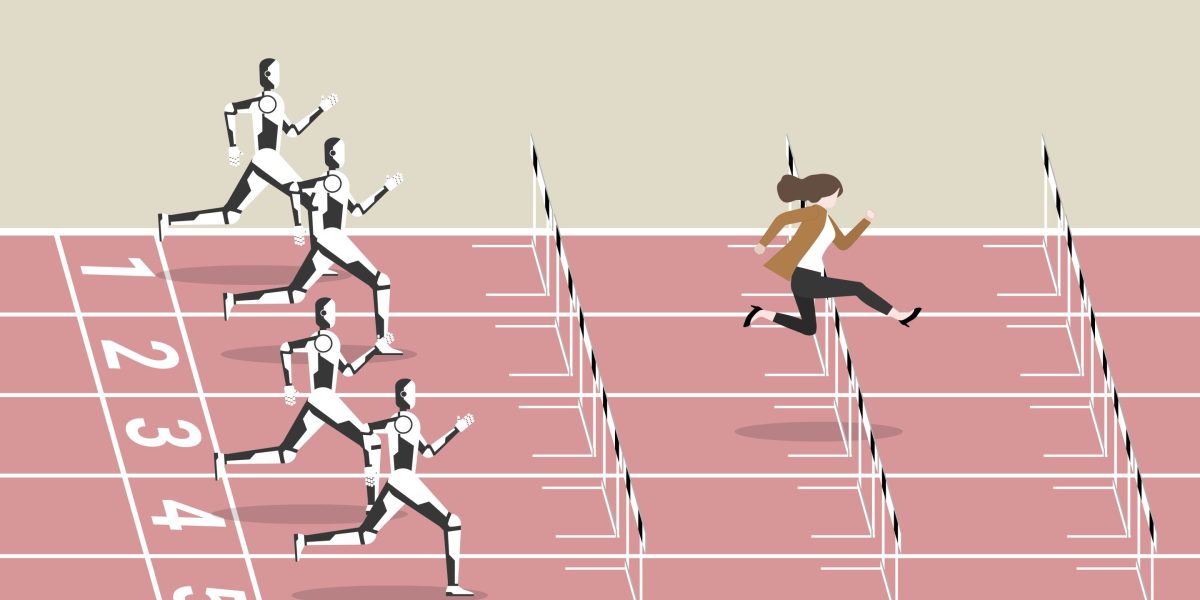
Every few months, a new large language model (LLM) is anointed AI champion, with record-breaking benchmark scores. But these celebrated metrics of LLM performance—such as testing graduate-level reasoning and abstract math—rarely reflect real business needs or represent truly novel AI frontiers. For companies in the market for enterprise AI models, basing the decision of which models to use on these leaderboards alone can lead to costly mistakes—from wasted budgets to misaligned capabilities and potentially harmful, domain-specific errors that benchmark scores rarely capture.
Public benchmarks can be helpful to individual users by providing directional indicators of AI capabilities. And admittedly, some code-completion and software-engineering benchmarks, like SWE-Bench or Codeforces, are valuable for companies within a narrow range of coding-related, LLM-based business applications. But the most common benchmarks and public leaderboards often distract both businesses and model developers, pushing innovation toward marginal improvements in areas unhelpful for businesses or unrelated to areas of breakthrough AI innovation.
The challenge for executives, therefore, lies in designing business-specific evaluation frameworks that test potential models in the environments where they’ll actually be deployed. To do that, companies will need to adopt tailored evaluation strategies to run at scale using relevant and realistic data.
The mismatch between benchmarks and business needs
The flashy benchmarks that model developers tout in their releases are often detached from the realities of enterprise applications. Consider some of the most popular ones: graduate-level reasoning (GPQA Diamond) and high school-level math tests, like MATH-500 and AIME2024. Each of these was cited in the releases for GPT o1, Sonnet 3.7, or DeepSeek’s R1. But none of these indicators is helpful in assessing common enterprise applications like knowledge management tools, design assistants, or customer-facing chatbots.
Instead of assuming that the “best” model on a given leaderboard is the obvious choice, businesses should use metrics tailored to their specific needs to work backward and identify the right model. Start by testing models on your actual context and data—real customer queries, domain-specific documents, or whatever inputs your system will encounter in production. When real data is scarce or sensitive, companies can craft synthetic test cases that capture the same challenges.
Without real-world tests, companies can end up ill-fitting models that may, for instance, require too much memory for edge devices, have latency that’s too high for real-time interactions, or have insufficient support for the on-premises deployment sometimes mandated by data governance standards.
Salesforce has tried to bridge this gap between common benchmarks and their actual business requirements by developing its own internal benchmark for its CRM-related needs. The company created its own evaluation criteria specifically for tasks like prospecting, nurturing leads, and generating service case summaries—the actual work that marketing and sales teams need AI to perform.
Reaching beyond stylized metrics
Popular benchmarks are not only insufficient for informed business decision-making but can also be misleading. Often LLM media coverage, including all three major recent release announcements, uses benchmarks to compare models based on their average performance. Specific benchmarks are distilled into a single dot, number, or bar.
The trouble is that generative AI models are stochastic, highly input-sensitive systems, which means that slight variations of a prompt can make them behave unpredictably. A recent research paper from Anthropic rightly argues that, as a result, single dots on a performance comparison chart are insufficient because of the large error ranges of the evaluation metrics. A recent study by Microsoft found that using a statistically more accurate clustered-based evaluation in the same benchmarks can significantly change the rank ordering of—and public narratives about—models on a leaderboards.
That’s why business leaders need to ensure reliable measurements of model performance across a reasonable range of variations, done at scale, even if it requires hundreds of test runs. This thoroughness becomes even more critical when multiple systems are combined through AI and data supply chains, potentially increasing variability. For industries like aviation or healthcare, the margin of error is small and far beyond what current AI benchmarks typically guarantee, such that solely relying on leaderboard metrics can obscure substantial operational risk in real-world deployments.
Businesses must also test models in adversarial scenarios to ensure the security and robustness of a model—such as a chatbot’s resistance to manipulation by bad actors attempting to bypass guardrails—that cannot be measured by conventional benchmarks. LLMs are notably vulnerable to being fooled by sophisticated prompting techniques. Depending on the use case, implementing strong safeguards against these vulnerabilities could determine your technology choice and deployment strategy. The resilience of a model in the face of a potential bad actor could be a more important metric than the model’s math or reasoning capabilities. In our view, making AI “foolproof” is an exciting and impactful next barrier to break for AI researchers, one that may require novel model development and testing techniques.
Putting evaluation into practice: Four keys to a scalable approach
Start with existing evaluation frameworks. Companies should start by leveraging the strengths of existing automated tools (along with human judgment and practical but repeatable measurement goals). Specialized AI evaluation toolkits, such as DeepEval, LangSmith, TruLens, Mastra, or ARTKIT, can expedite and simplify testing, allowing for consistent comparison across models and over time.
Bring human experts to the testing ground. Effective AI evaluation requires that automated testing be supplemented with human judgment wherever possible. Automated evaluation could include a comparison of LLM answers to ground truth answers, or the use of proxy metrics, such as automated ROUGE or BLEU scores, to gauge the quality of text summarization.
For nuanced assessments, however, ones where machines still struggle, human evaluation remains vital. This could include domain experts or end-users conducting a “blind” review of a sample of model outputs. Such actions can also flag potential biases in responses, such as LLMs giving responses about job candidates that are biased by gender or race. This human layer of review is labor-intensive, but can provide additional critical insight, like whether a response is actually useful and well-presented.
The value of this hybrid approach can be seen in a recent case study where a company evaluated an HR-support chatbot using both human and automated tests. The company’s iterative internal evaluation process with human involvement showed a significant source of LLM response errors was due to flawed updates to enterprise data. The discovery highlights how human evaluation can uncover systemic issues beyond the model itself.
Focus on tradeoffs, not isolated dimensions of assessment. When evaluating models, companies must look beyond accuracy to consider the full spectrum of business requirements: speed, cost efficiency, operational feasibility, flexibility, maintainability, and regulatory compliance. A model that performs marginally better on accuracy metrics might be prohibitively expensive or too slow for real-time applications. A great example of this is how Open AI’s GPT o1(a leader in many benchmarks at release time) performed when applied to the ARC-AGI prize. To the surprise of many, the o1 model performed poorly, largely due to ARC-AGI’s “efficiency limit” on the computing power used to solve the benchmark tasks. The o1 model would often take too long, using more compute time to try to come up with a more accurate answer. Most popular benchmarks don’t have a time limit even though time would be a critically important factor for many business use cases.
Tradeoffs become even more important in the growing world of (multi)-agentic applications, where simpler tasks can be handled by cheaper, quicker models (overseen by an orchestration agent), while the most complex steps (such as solving the broken-out series of problems from a customer) could need a more powerful version with reasoning to be successful.
Microsoft Research’s HuggingGPT, for example, orchestrates specialized models for different tasks under a central language model. Being prepared to change models for different tasks requires building flexible tooling that isn’t hard-coded to a single model or provider. This built-in flexibility allows companies to easily pivot and change models based on evaluation results. While this may sound like a lot of extra development work, there are a number of available tools, like LangChain, LlamaIndex, and Pydantic AI, that can simplify the process.
Turn model testing into a culture of continuous evaluation and monitoring. As technology evolves, ongoing assessment ensures AI solutions remain optimal while maintaining alignment with business objectives. Much like how software engineering teams implement continuous integration and regression testing to catch bugs and prevent performance degradation in traditional code, AI systems require regular evaluation against business-specific benchmarks. Similar to the practice of pharmacovigilance among users of new medicines, feedback from LLM users and affected stakeholders also needs to be continuously gathered and analyzed to ensure AI “behaves as expected” and doesn’t drift from its intended performance targets.
This kind of bespoke evaluation framework fosters a culture of experimentation and data-driven decision-making. It also enforces the new and critical mantra: AI may be used for execution, but humans are in control and must govern AI.
Conclusion
For business leaders, the path to AI success lies not in chasing the latest benchmark champions but in developing evaluation frameworks for your specific business objectives. Think of this approach as “a leaderboard for every user,” as one Stanford paper suggests. The true value of AI deployment comes from three key actions: defining metrics that directly measure success in your business context; implementing statistically robust testing in realistic situations using your actual data and in your actual context; and fostering a culture of continuous monitoring, evaluation and experimentation that draws on both automated tools and human expertise to assess tradeoffs across models.
By following this approach, executives will be able to identify solutions optimized for their specific needs without paying premium prices for “top-notch models.” Doing this can hopefully help steer the model development industry away from chasing marginal improvements on the same metrics—falling victim to Goodhart’s law with capabilities of limited use for business—and instead free them up to explore new avenues of innovation and the next AI breakthrough.
Read other Fortune columns by François Candelon.
Francois Candelon is a partner at private equity firm Seven2 and the former global director of the BCG Henderson Institute.
Theodoros Evgeniou is a professor at INSEAD and a cofounder of the trust and safety company Tremau.
Max Struever is a principal engineer at BCG-X and an ambassador at the BCG Henderson Institute.
David Zuluaga Martínez is a partner at Boston Consulting Group and an ambassador at the BCG Henderson Institute.
Some of the companies mentioned in this column are past or present clients of the authors’ employers.
This story was originally featured on Fortune.com
Source link
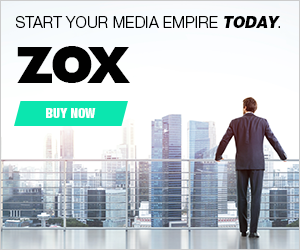

Boux Avenue sales still falling but it expects improvements this year

Buy-now-pay-later installment plans will now appear on your credit report

UK retail sales sluggish in March says BDO report, but fashion starts to bounce back
Trending
-
Entertainment8 years ago
The final 6 ‘Game of Thrones’ episodes might feel like a full season
-
Politics8 years ago
Congress rolls out ‘Better Deal,’ new economic agenda
-
Entertainment8 years ago
Mod turns ‘Counter-Strike’ into a ‘Tekken’ clone with fighting chickens
-
Entertainment8 years ago
New Season 8 Walking Dead trailer flashes forward in time
-
Tech8 years ago
Microsoft Paint is finally dead, and the world Is a better place
-
Tech8 years ago
Hulu hires Google marketing veteran Kelly Campbell as CMO
-
Tech8 years ago
Ford’s 2018 Mustang GT can do 0-to-60 mph in under 4 seconds
-
Politics8 years ago
Illinois’ financial crisis could bring the state to a halt